COVID-19 Trend Forecasting Using State-level Mobility and Policy
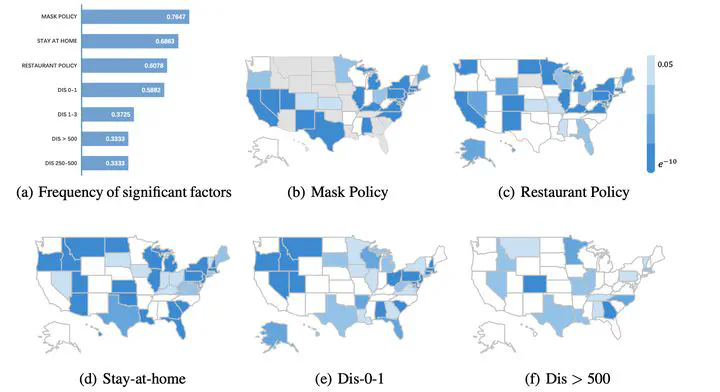
Abstract
The importance of pandemic forecast cannot be overemphasized. We propose an interpretable machine learning approach for forecasting pandemic transmission rates by utilizing local mobility statistics and government policies. A calibration step is introduced to deal with time-varying relationships between transmission rates and predictors. Experimental results demonstrate that our approach is able to make accurate two-week ahead predictions of the state-level COVID-19 infection trends in the US. Moreover, the models trained by our approach offer insights into the spread of COVID-19, such as the association between the baseline transmission rate and the state-level demographics, the effectiveness of local policies in reducing COVID-19 infections, and so on. This work provides a good understanding of COVID-19 evolution with respect to state-level characteristics and can potentially inform local policymakers in devising customized response strategies.
Type
Publication
medRxiv